Scaling Smartly: Mitigating Risks in AI Adoption with Micro-Deployments
- Jared Smith
- Aug 18, 2024
- 5 min read
When Big Dreams Hit Small Realities
Ah, the dream of AI—where machines work tirelessly, the business hums along smoothly, and employees find themselves freed from mundane tasks to focus on innovation. That’s the pitch, right? But somewhere between the boardroom presentations and the real-world rollout, things often go awry. You’ve seen it before: a massive investment in an enterprise-level AI system that’s supposed to revolutionize your industry, only for it to become the corporate equivalent of a treadmill—lots of motion, no forward progress.
Why does this happen? The reality is that many large-scale AI implementations miss the most critical factor for success: the people who actually have to use the technology. When AI is introduced from the top down without considering the day-to-day realities of your employees, it’s like giving them a Ferrari without any driving lessons—sure, it’s a powerful tool, but who knows how to use it without crashing?
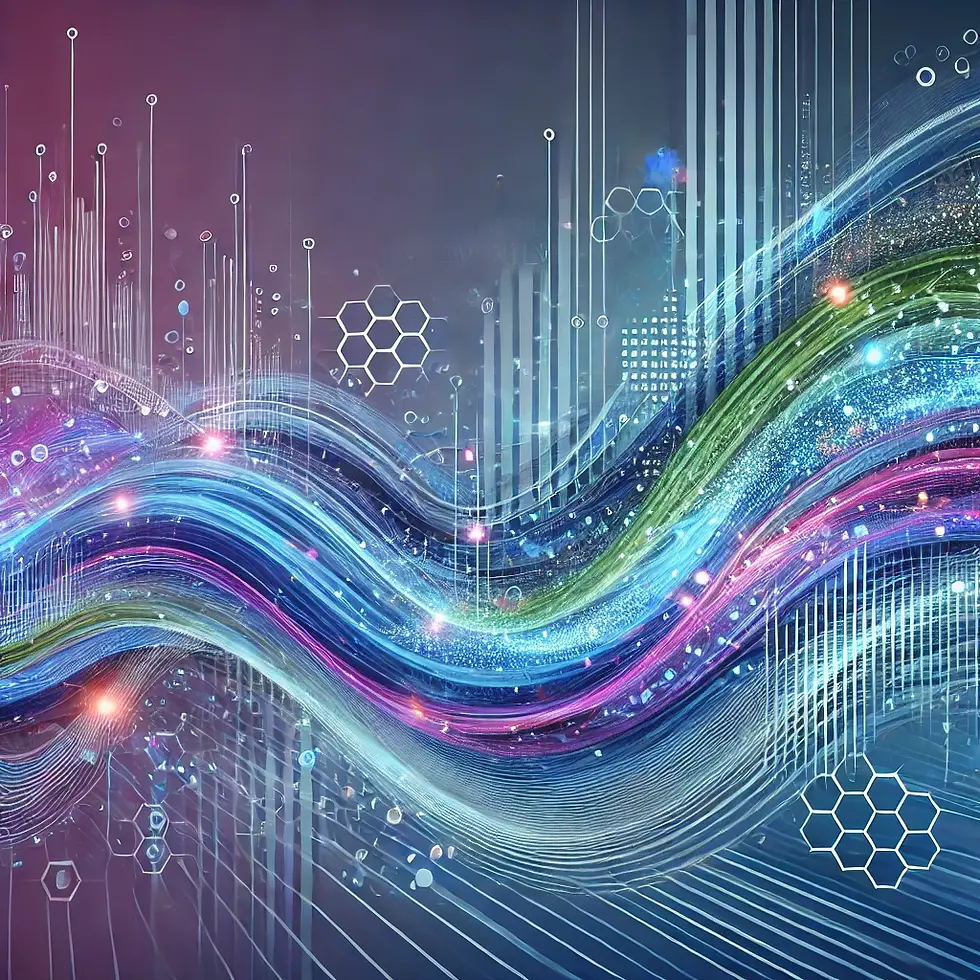
The Risks of Going Big: When More Isn’t Better
Let’s talk risks. First up, there’s the financial sinkhole. Enterprise-level AI systems don’t come cheap. We’re talking tens of millions of dollars just to get these systems off the ground. And while the promise of high returns can be alluring, it often blindsides decision-makers to the actual costs and complexities involved. It’s like betting big in Vegas—everyone loves the idea of hitting the jackpot, but the odds? Not so much in your favor.
But even more concerning is the misalignment with employee needs. These AI tools, despite their sophistication, often don’t address the real pain points of the people on the ground. Imagine rolling out a cutting-edge AI tool to help your sales team, only to find they’re using it less than the office microwave. The reason? The AI doesn’t speak their language, doesn’t integrate with their workflow, or simply isn’t necessary. It’s a classic case of innovation for innovation’s sake, rather than a tool that actually helps.
And then there’s the innovation trap. Companies, eager to stay ahead of the curve, rush into AI adoption without a clear understanding of how it fits into their existing ecosystem. The result? An over-hyped solution that’s underutilized—another shiny object collecting dust while employees stick with their tried-and-true methods.
Micro-Deployments: Small, but Mighty
So, what’s the alternative? Enter micro-deployments. Think of them as the MVPs (Minimum Viable Products) of AI integration. Instead of launching a full-scale AI solution across your entire enterprise, you start small—really small. Pick an isolated use case, one where you can test the waters without the risk of a financial tsunami.
What’s the magic in this approach? First, you lower your risks. With micro-deployments, you’re not betting the farm; you’re planting a seed. If it grows, great—scale it up. If it doesn’t, you’ve lost a sapling, not an orchard.
Second, micro-deployments allow for faster iteration. When you’re working on a small scale, feedback loops are quicker, and you can refine your AI tool based on real-world usage rather than theoretical models. It’s like beta testing with real users—except these users are your employees, and their buy-in is critical.
Finally, successful micro-deployments act as proof of concept. They provide tangible evidence that your AI can deliver value, making it easier to justify broader rollouts. Plus, they create internal momentum—employees see the benefits firsthand, making them more likely to champion the technology when it’s scaled.
Building the Buzz: The Secret Sauce to Adoption
Here’s where things get interesting. Remember how I mentioned that internal momentum? That’s your secret weapon for broader adoption. When employees see AI solving real problems in their corner of the company, word spreads. It’s like the office equivalent of a viral meme—except this one actually improves productivity.
Creating hype around micro-deployments isn’t just about showing off the technology. It’s about involving employees from the get-go. User-centered design isn’t just a buzzword; it’s the backbone of successful AI adoption. When employees feel like they’ve had a hand in shaping the tools they use, they’re more likely to embrace them. And when they embrace them, they become your internal champions, spreading the gospel of AI throughout the organization.
But don’t just stop at hype. Build a culture where early adopters are recognized and rewarded. These are the folks who will help ease the transition when it’s time to roll out AI on a larger scale. They’re your first wave of advocates, and their enthusiasm can make or break your broader strategy.
### Case in Point: Micro-Deployments in Action
Let’s take a hypothetical trip to a mid-sized construction company—let’s call it “BuildSmart.” They’ve heard all about AI and are eager to jump on the bandwagon, but their CFO is wary of dropping millions on an unproven technology. So, they start with a micro-deployment—a small AI tool designed to optimize project scheduling on just one site.
The results? Impressive. The AI tool not only streamlines scheduling but also uncovers inefficiencies that manual methods missed. Word gets out, and soon other project managers are clamoring to get the tool for their sites. The buzz builds, and before you know it, BuildSmart has a groundswell of support for a company-wide AI rollout—one that’s now been tested, refined, and proven.
Rogue Wave’s Approach: Micro-Deployments at the User Level
At Rogue Wave, we practice what we preach. Our approach to AI integration is grounded in the principles of micro-deployment. We start at the user level, developing custom AI solutions that address specific challenges within small, isolated teams. This strategy allows us to test and refine our tools in real-world scenarios, ensuring they meet the needs of the end-users before we consider scaling.
But don’t just take our word for it—listen to what our clients are saying:
“I contacted Roguewave.ai as I knew we needed private AI capability for our consulting business but didn’t have time to experiment and figure it out on my own. Within an hour, the private LLM was up with basic configuration in place. A couple of more hours of ‘teaching’ our methodology to the LLM, and we were off to the races. On the first day, I’d conservatively estimate that we reduced 160 hours of deep analytical work to 1-2 hours. If you’re ready to be blown away, get in touch with Roguewave.ai.”
This testimonial highlights the power of micro-deployments. By focusing on a specific use case—implementing a private large language model (LLM) for a consulting business—we were able to deliver rapid results that far exceeded expectations. Within hours, our client was leveraging AI to drastically reduce the time required for deep analytical work. This not only proved the effectiveness of the tool but also generated immediate excitement and buy-in from the team.
By employing micro-deployments, we create a buzz within the organization that drives broader adoption. Employees see the immediate benefits of the AI tools we develop, leading to organic growth and excitement about future rollouts. This bottom-up approach not only mitigates the risks associated with large-scale AI implementations but also ensures that the technology is embraced by those who will use it daily.
Roadmap to Success: Implementing Micro-Deployments
Ready to take the plunge? Here’s how to do it right:
1. Identify High-Impact Use Cases: Look for areas where AI can make a real difference quickly—think of pain points that have a clear ROI.
2. Involve End-Users from the Start: Design with your employees in mind. Get their input early, and make sure the AI is solving their problems, not creating new ones.
3. Pilot and Iterate: Start small, gather feedback, and refine the tool. Don’t rush to scale—let the micro-deployment run its course and make improvements as needed.
4. Leverage Success to Scale: Use the momentum from your successful micro-deployment to drive broader adoption. Celebrate wins, and let your early adopters lead the charge.
Smart Scaling for Sustainable AI Adoption
In the end, the goal isn’t just to implement AI—it’s to make it stick. By starting with micro-deployments, you not only mitigate the risks associated with large-scale rollouts but also build a solid foundation for future success. Remember, it’s not about going big; it’s about going smart. With the right approach, you can turn AI from a daunting investment into a powerful tool that truly transforms your organization.
Comments